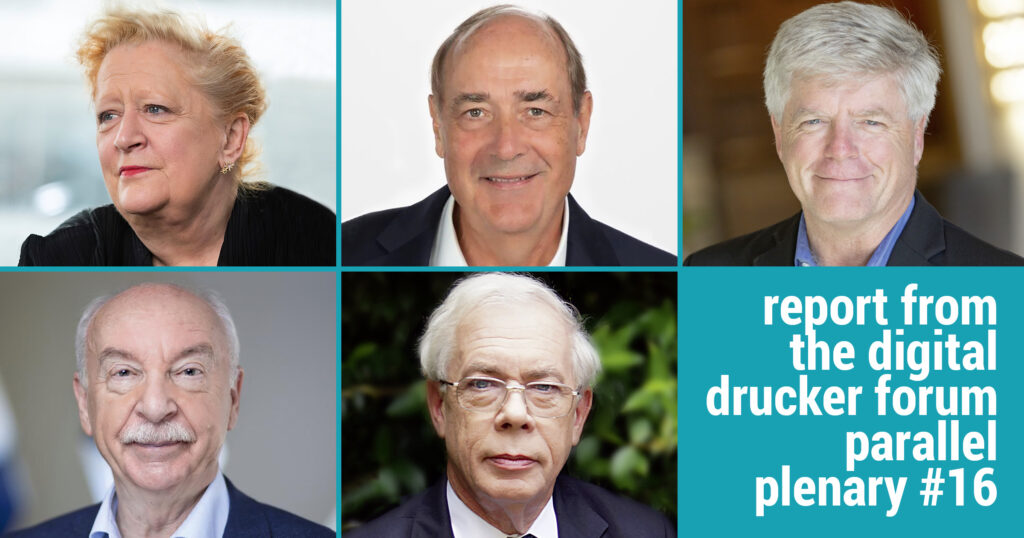
Moderator
Margaret Heffernan Entrepreneur; Professor of Practice, University of Bath School of Management, England
Speakers
Paul J. H. Schoemaker Educator, researcher and entrepreneur in strategic management, decision making, innovation and leadership
Tom Davenport Distinguished Professor of Information Technology and Management, Babson College; Senior Advisor to Deloitte U.S.
Gerd Gigerenzer Director of the Harding Center for Risk Literacy, University of Potsdam
John Kay Economist; former Chair at LSE, LBS and Oxford
In intensely competitive markets, many decision processes are being sped up or handed over to number-crunching machines. Where should we still be deeply deliberative? Four excellent panellists ably moderated by Margaret Heffernan were invited to discuss this very relevant topic.
Margaret Heffernan introduced the topic by stating that we know that life is always uncertain, but management wants to obliterate uncertainty which it tries to do by forecasting, planning, and executing. But this comes with no certainty.
Managers want to maintain the status quo but have yet to realise that the status quo is uncertainty. She goes on to say it is also at the edges of the known that opportunities exist.
What do we think we know?
Paul J.H. Schoemaker Whether it is uncertainty, risk, ambiguity or ignorance depends on what we think or think we know. He introduced Shell as an example, in particular their engineering, a lot of technocrats, but who embrace humility and what they don’t know as their way of dealing with uncertainty. It’s recognised that people tend to be overconfident in their predictive abilities, show biases and pretend they know. A big difference is how lay people think what is uncertain and what they perceive as risk, depending on how well they understand it. A focus might move to what could happen but without too much detail, so a series of possible futures, rather than one fixed one.
Where algorithms work and where they don’t
It’s wrong to assume that artificial intelligence will overpower our human intelligence, says Gerd Gigerenzer. Algorithms work in stable, well-defined environments but don’t work well when human behaviour interacts. He adds that risk is a situation when we know all possibilities; uncertainty is where we normally live. Life is not a simple risk based issue, like the Lotto where you can calculate the chances. Risk based scenarios inhibit innovation and discovery. Most economic models are constructed on this basis. Most behavioural economists also evaluate human judgement along these lines, and deviations from the model are labelled as biases.
Tools to deal with uncertainty
He proposes some tools to deal with uncertainty. Scenario planning is a possibility but equally difficult in conditions of uncertainty, as it is based on the past. Heuristics is another tool, which ignores the past and complex models and looks for the variables powerful enough to give some clue towards the future. If there is risk then use models and big data but if the situation is one of uncertainty, then he greater reliance on recent data and heuristics.
In this writer’s experience SMEs are particularly good at this. They run heuristics as a matter of course as they tend not to have enough big data or resources. Often, they are running heuristics unknowingly which produces its own problems, but overall, they are more flexible, agile, and resilient than larger businesses. This is partly based on their ability to make fast decisions, and are therefore more sustainable as a business. For big businesses, the Rendanheyi and similar models may offer some solutions.
Risk or uncertainty?
The question has to be is this a risk or is it an uncertainty. Gigerenzer worked with the Bank of England on simple decision trees to predict bank failures using heuristics rather than the risk models of ever-increasing complexity in Basel II and Basel III (the international banking regulatory accords) which failed to predict failures. His view is that complexity can be a self defence mechanism whereas a fast and frugal approach generates more transparency. So much so that a single data point can be better than big data.
AI’s role in uncertainty
Tom Davenport, steeped in AI, argues that it can play a role in uncertainty. It is acknowledged that AI is not well suited to decision making under uncertainty, because machine learning is good at tactical repetitive decisions based on big data but has little use where human intuition is involved. Tom is not comfortable just using human intuition but is frustrated by a lot of scenario planning, as too many human interventions make the model too subjective. He’s comfortable using AI and heuristics together on the assumption that the rules part produces some structure to the debate. Anticipating the future still has some AI validity to give us alerts on a large scale, where the human element may not have enough scope or data. But he still acknowledges that a human decision based on informed intuition is necessary to interpret the data outputs.
Over reliance on behavioural economics
John Kay is not a big fan of behavioural economics as he argues it’s difficult to predict the future or attach some probability when most issues are unique, and can be vague or ambiguous, some even after the event. His alternative is not unaided intuition but decisions based on judgement and experience. Behavioural economics has its strengths in determining what people actually do rather than producing models on what a rational actor ought to do. But it has morphed into attempting to determine how the world works and if people don’t conform to the model, it is the fault of the people who need to be knocked into shape so that the model works. The mistakes from BE are that most are unique problems, such that no model will fit. Our decisions, so called mistakes, are in fact adaptive responses to a changing environment and are not often fully understood, even after the event. He suggests a more useful approach would be to recognise that there are mainly vague and uncertain outcomes. Therefore, do not attempt to produce a prediction of the future, but identify risks that construct narratives which are robust and resilient to minimise issues and maximise outcomes.
Summary
Use an adaptive toolbox.
Check heuristics by having a diverse group which should reduce biases.
Use heuristics, AI, and scenario planning appropriately, recognising that uncertainty is certain, and it is where innovation and opportunities arise.
Where there is a critical issue, buffers (e.g additional stock etc) may be needed, so moving away from Just-in-Time management
We need to feel a little less comfortable in expecting more of the same. Remember that models are only guides to think about the present and future, not strict representations of what will happen. Emphasising optimisation and efficiency, the usual role of management, may not be helpful as the thinking is too brittle. A more modular system with some redundancy built in should give businesses better resilience. An efficient business has built in risks, and there is little robustness in general management thinking. (Resilient being able to recover from a problem, robust means avoiding the problem).
Last questions
Does building a robust business mean that it has less agility? Should we aim for more agility and resilience instead? Should this be a binary question, or perhaps should some parts of the business be robust, but less agile, and others resilient but more agile?
About the Author:
Nick Hixson is an entrepreneur, business enabler based in the South of England, and Drucker Associate